New AI Model Predicts Ovarian Cancer Therapy Success with 80% Accuracy
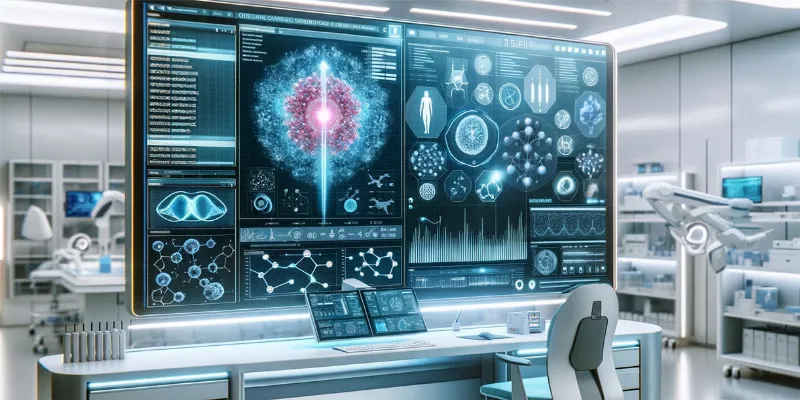
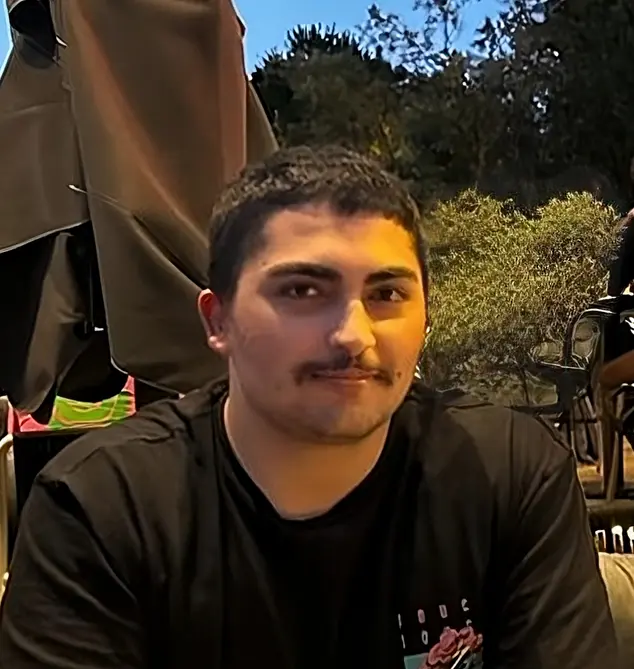
Digital Health |
20 November 2023
Newly developed artificial intelligence model, named IRON AI, could revolutionize personalized ovarian cancer therapy by predicting treatment responses with 80% accuracy. The groundbreaking results of this study, published in Nature Communications, have the potential to transform current treatment models and improve success rates. This AI tool surpasses existing methods by analyzing comprehensive patient data to accurately forecast treatment outcomes.
The AI model, known as Integrated Radiogenomics for Ovarian Neoadjuvant therapy (IRON), was developed by Professor Evis Sala and his team at the University of Cambridge. This significant advancement is the result of a study conducted on 134 patients with high-grade serous ovarian carcinoma (HGSOC). The AI-based prediction model has demonstrated a remarkable accuracy rate of 80% in forecasting therapy outcomes for these patients.
Ovarian cancer annually impacts over 100,000 women globally, often diagnosed late due to vague early symptoms. High-grade serous ovarian carcinoma, making up 70-80% of cases, is notably aggressive and often resistant to chemotherapy. Current prediction methods for treatment response are only 50% accurate. The cancer's high heterogeneity limits effective biomarkers, prompting the creation of an AI tool for more accurate chemotherapy response prediction.
The IRON Model: A Fusion of Data for Precision Medicine
"We compiled two independent datasets with a total of 134 patients (92 cases in the first dataset, 42 in the second independent test set)," Professor Sala and Dr. Mireia Crispin Ortuzar from Cambridge explained. For each patient, clinicians collected comprehensive clinical data, encompassing demographic information and treatment details, as well as blood biomarkers such as CA-125 and circulating tumor DNA (ctDNA).
By analyzing these diverse data sources, IRON AI accurately predicts changes in total disease volume at diagnosis, offering a new level of precision in treatment planning.
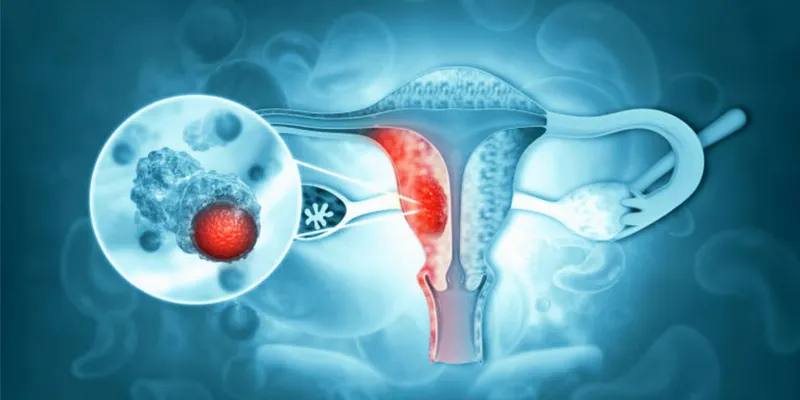
The Study's Findings: Radiomics and Biomarkers in Focus
The study's extensive data analysis, conducted on 134 patients, revealed several key insights:
- Response Patterns: Omental and pelvic/ovarian tumor locations, common in ovarian cancer, showed significant differences in response to neoadjuvant chemotherapy (NACT). Omental tumors, in particular, responded better compared to pelvic tumors.
- Biomarkers: Baseline levels of CA-125 and TP53 mutant allele fraction (MAF) exhibited strong correlations with disease burden. Interestingly, while these markers didn't correlate with treatment response, they showed a significant relationship with post-chemotherapy disease measurements.
- Radiomics Features: The researchers identified six distinct clusters of imaging features, each correlating with specific biological or clinical factors. This highlights the potential of radiomics in contributing unique insights into disease extent, spread, and heterogeneity.
Implications for Clinical Practice and Research
The IRON framework's high accuracy in predicting treatment response represents a significant stride forward in personalizing therapy for HGSOC. This model could greatly impact patient stratification in clinical trials, potentially avoiding surgery delays for non-responsive patients and facilitating the discovery of novel therapies.
Combining AI with Clinical Expertise
Professor Sala's visionary approach combines AI's analytical power with clinical expertise, offering a more nuanced understanding of HGSOC. This methodology addresses previous criticisms of radiomics regarding robustness and biological interpretability, demonstrating that carefully designed models can yield highly reliable and clinically relevant insights.
A New Era in Ovarian Cancer Treatment
The integration of AI in predicting therapy outcomes for ovarian cancer marks a new era in personalized medicine. By harnessing the power of diverse data sets, the IRON model offers hope for more effective and tailored treatments for ovarian cancer patients, potentially transforming the landscape of cancer care and research.
Abstract of the research
Integrated radiogenomics models predict response to neoadjuvant chemotherapy in high grade serous ovarian cancer
Abstract: High grade serous ovarian carcinoma (HGSOC) is a highly heterogeneous disease that typically presents at an advanced, metastatic state. The multi-scale complexity of HGSOC is a major obstacle to predicting response to neoadjuvant chemotherapy (NACT) and understanding critical determinants of response. Here we present a framework to predict the response of HGSOC patients to NACT integrating baseline clinical, blood-based, and radiomic biomarkers extracted from all primary and metastatic lesions. We use an ensemble machine learning model trained to predict the change in total disease volume using data obtained at diagnosis (n = 72). The model is validated in an internal hold-out cohort (n = 20) and an independent external patient cohort (n = 42). In the external cohort the integrated radiomics model reduces the prediction error by 8% with respect to the clinical model, achieving an AUC of 0.78 for RECIST 1.1 classification compared to 0.47 for the clinical model. Our results emphasize the value of including radiomics data in integrative models of treatment response and provide methods for developing new biomarker-based clinical trials of NACT in HGSOC.
Comments
No Comments Yet!