Machine Learning Could Detect Disease from Proteins 15 Years Before It Strikes
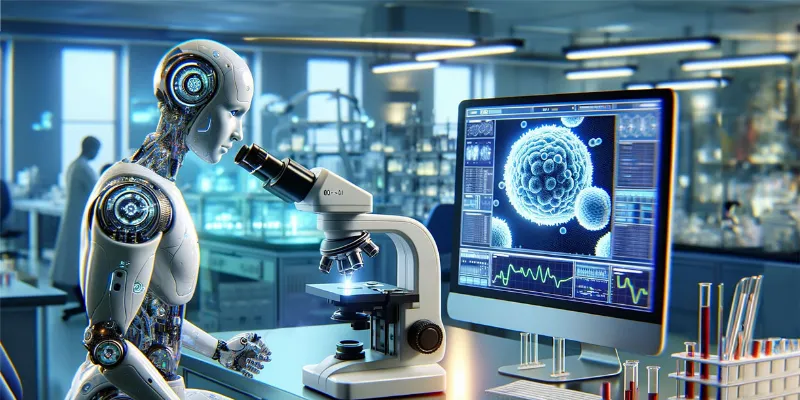
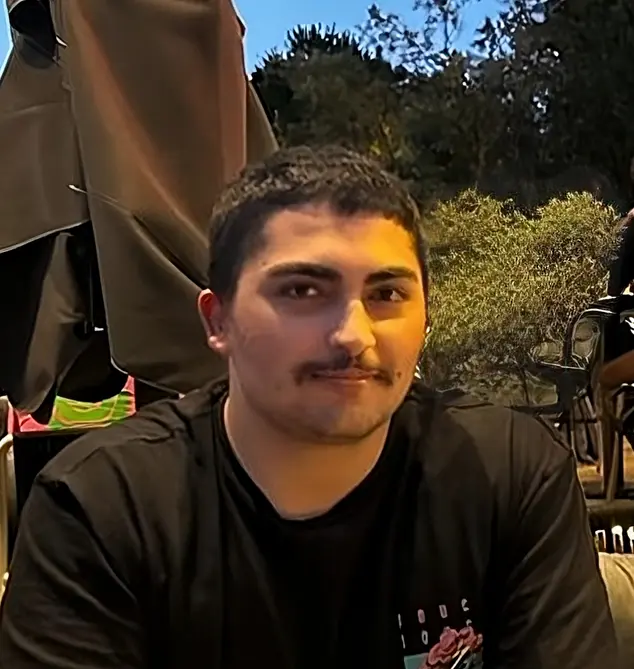
Digital Health |
15 July 2024
New predictive models using machine learning to analyze protein patterns from UK Biobank data could predict disease risks, including Alzheimer's, diabetes, and heart disease, up to 15 years in advance. This breakthrough has the potential to transform early detection and prevention strategies in healthcare.
Researchers from Optima Partners, Biogen, and the University of Edinburgh have made a significant breakthrough in disease risk prediction. Published in the prestigious journal Nature Aging, the research highlights a groundbreaking approach that uses machine learning to analyze protein patterns in blood, offering a window into a patient’s future health.
The Study's Core Insights
The research focused on analyzing a vast array of medical data from the UK Biobank, particularly examining the correlations between 1,468 Olink protein levels and 23 age-related diseases and mortality. A cohort of 47,600 participants, who provided blood samples from 2006 to 2010, was scrutinized to forecast disease risks up to 15 years in advance.
Among the notable findings was the association of protein GDF15, a marker of inflammation, with a range of conditions including Alzheimer’s, vascular dementia, heart disease, liver disease, type 2 diabetes, and all-cause mortality.
Implications for Healthcare
The study's implications are vast and profound. "Our research represents a promising step forward in risk prediction," said Dr. Danni Gadd, the first author of the study. "It’s encouraging to see how much potential there is from a single blood sample that allow us to predict a range of disease outcomes. Being able to detect early warning signs for a broad set of conditions may lead to opportunities for early intervention and prevention, marking a significant moment for the healthcare industry.”
Advanced Predictive Models
Researchers developed what they call "ProteinScores," utilizing penalized Cox regression to enhance prediction accuracy. These scores improved the area under the curve estimates for predicting disease onset within 10 years, surpassing traditional methods that include age, sex, lifestyle factors, and other biomarkers. Notably, the ProteinScore for type 2 diabetes outperformed both the polygenic risk score and HbA1c tests, which are commonly used to monitor and diagnose the disease.
Dr. Chris Foley, one of the principal investigators, chief scientist of Optima Partners, cautioned that more work is needed before these findings can be routinely used in clinical settings. "However, our discoveries set strong foundations for the inclusion of new risk prediction signatures to shed a light on possible pathways and mechanisms that underlie diseases. Pattern recognition like this would not be possible without modern machine learning technology and its capacity to analyse data at this scale and will in turn allow us to address some of the most pressing healthcare challenges of our time," said Dr. Foley.
This breakthrough, enabled by advanced machine learning, marks a significant advancement in healthcare, offering a promising avenue for more precise and personalized treatment plans.
From the Research
Researchers analyzed 1,468 Olink protein levels from 47,600 UK Biobank participants, identifying 3,209 associations linked to 21 age-related diseases and mortality. They developed "ProteinScores" using penalized Cox regression, which, in tests, enhanced predictions of disease onset over 10 years, outperforming traditional metrics like age, sex, lifestyle factors, and biomarkers. Notably, the ProteinScore for type 2 diabetes surpassed both polygenic risk scores and HbA1c tests, highlighting the plasma proteome's potential in disease risk stratification.
Comments
No Comments Yet!