Scientists Have Developed Next-Generation Drug with AI Collaboration
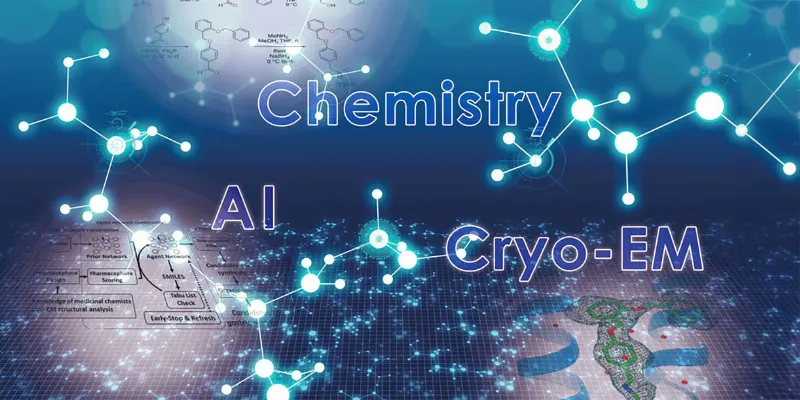
30 October 2023
Scientists at Nagoya University in Japan have utilized artificial intelligence (AI) to develop a next-generation gastric acid inhibitor that displays a significantly higher binding affinity than current medications. This revelation suggests a dynamic shift in the development of pharmaceuticals by pairing human expertise with AI.
A research team led by Associate Professor Kazuhiro Abe and Professor Satoshi Yokoshima collaborated with Intage Healthcare Corporation and the SPring-8 radiation facility. Their focus was the intricate structure of the gastric proton pump, a protein responsible for transporting the acid-producing H+ protons.
Collaborating with "Deep Quartet", an AI-driven drug discovery platform, they synthesized a compound, DQ-18, that binds nearly ten times stronger than existing inhibitors. The goal was to discover compounds that could bind at several sites on the proton pump, increasing the drug's overall efficacy. These compounds were then synthesized and their protein binding structures analyzed through cryo-electron microscopy. The team further enhanced the compounds' binding ability based on these analyses.
Remarkably, the AI generated over 100 distinct chemical structures as potential inhibitors. Following rigorous testing, the sixth synthesized compound, DQ-06, showcased a superior binding ability compared to existing reference drugs. The researchers didn't stop there. Using insights from cryo-electron microscopy, they identified areas for improvement in the binding strength. This led to the creation of another compound, DQ-18, by introducing a chlorine atom into DQ-06, resulting in an even stronger bond.
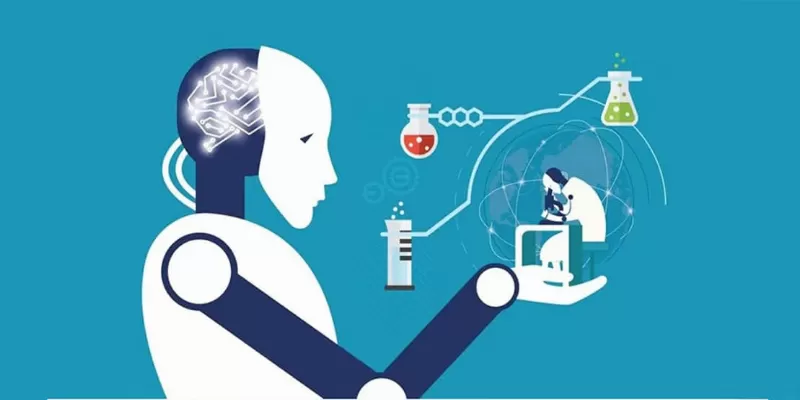
The outcome was impressive. The new compound exhibited a binding affinity nearly ten times greater than SCH28080, a prototype for gastric acid inhibitors. Associate Professor Kazuhiro Abe expressed his belief in the power of human and AI collaboration, stating,
"We can see AI being useful for creating treatments, but not completely or automatically. We used AI for structure-based drug design, which we humans are not so good at. But we chose real candidates to synthesize, and indeed we improved it with our own hands. We efficiently used AI for what we are not good at.”
This groundbreaking research sets a new precedent in pharmaceutical development, heralding more effective treatments for acid-related conditions. It also underscores the transformative potential of AI in the medical field, not as a replacement, but as a collaborative force alongside human experts.
Abstract of the research
Deep learning driven de novo drug design based on gastric proton pump structures
Abstract: Existing drugs often suffer in their effectiveness due to detrimental side effects, low binding affinity or pharmacokinetic problems. This may be overcome by the development of distinct compounds. Here, we exploit the rich structural basis of drug-bound gastric proton pump to develop compounds with strong inhibitory potency, employing a combinatorial approach utilizing deep generative models for de novo drug design with organic synthesis and cryo-EM structural analysis. Candidate compounds that satisfy pharmacophores defined in the drug-bound proton pump structures, were designed in silico utilizing our deep generative models, a workflow termed Deep Quartet. Several candidates were synthesized and screened according to their inhibition potencies in vitro, and their binding poses were in turn identified by cryo-EM. Structures reaching up to 2.10 Å resolution allowed us to evaluate and re-design compound structures, heralding the most potent compound in this study, DQ-18 (N-methyl-4-((2-(benzyloxy)-5-chlorobenzyl)oxy)benzylamine), which shows a Ki value of 47.6 nM. Further high-resolution cryo-EM analysis at 2.08 Å resolution unambiguously determined the DQ-18 binding pose. Our integrated approach offers a framework for structure-based de novo drug development based on the desired pharmacophores within the protein structure.
Comments
No Comments Yet!