AI in Life Sciences: Trends and Predictions for 2025 - By Doron Sitbon
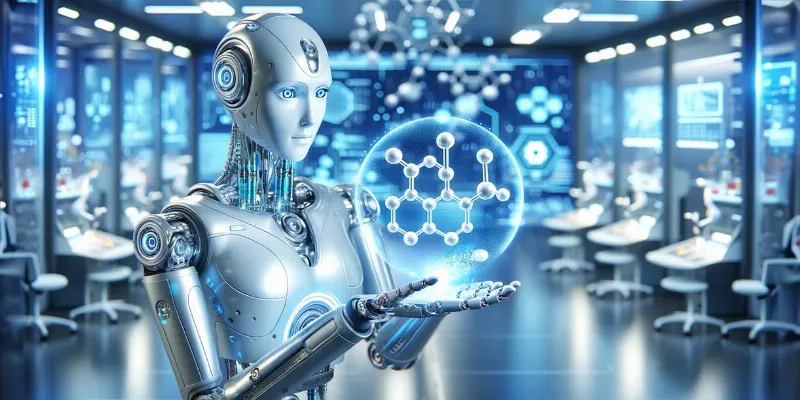
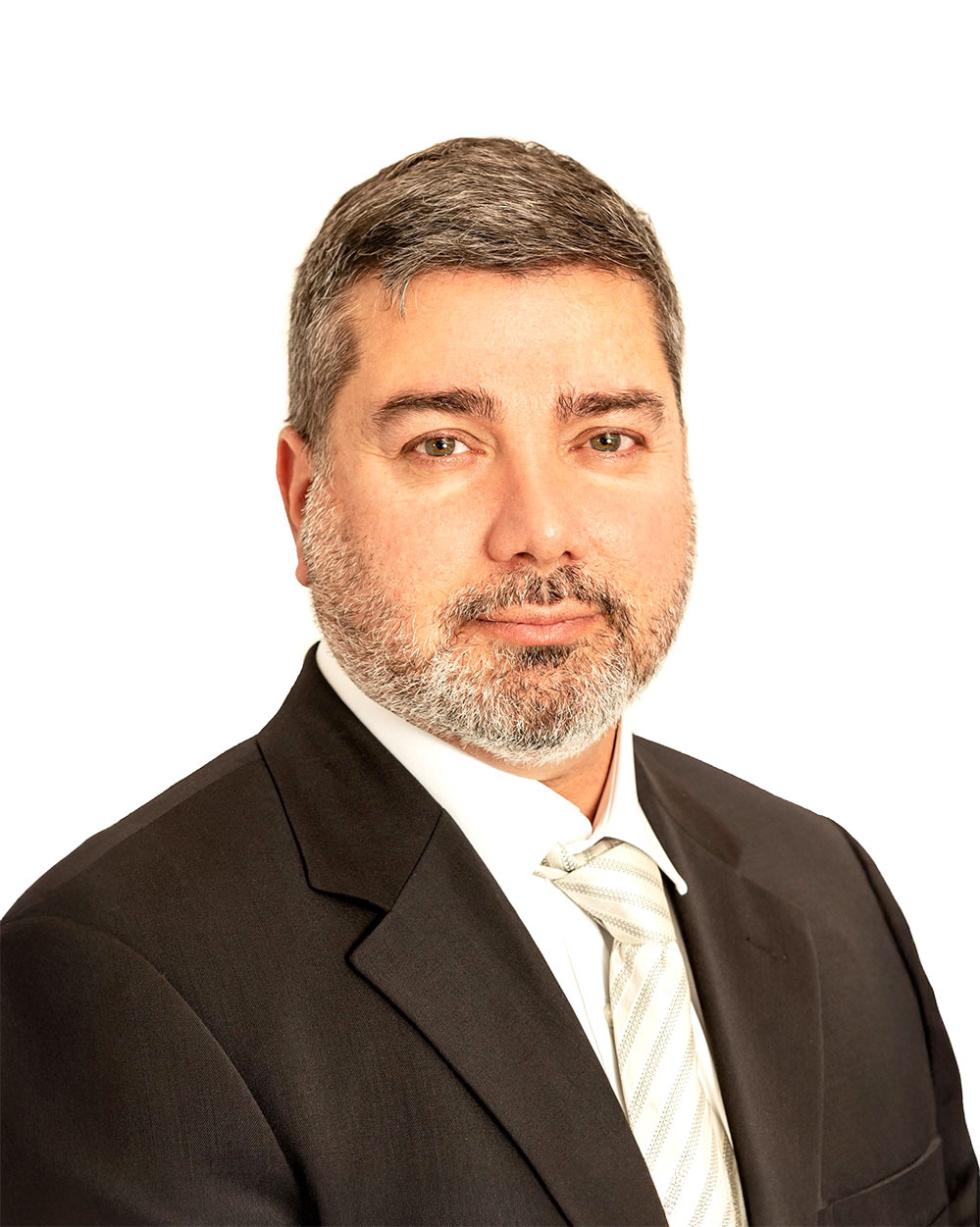
Artificial intelligence (AI) is evolving fast and life sciences is feeling the impact. In 2025, its role will expand even further, driving efficiencies and accelerating discoveries. The industry has already seen what AI can do but realizing its full potential comes with new challenges—governance, emerging regulations and the push for real-world value. And the shift is already happening. Recent AI breakthroughs are setting the stage for what happens next.
The AI Arms Race and a New Economic Equilibrium
We’ve barely set foot in 2025 yet Chinese tech companies DeepSeek and Alibaba have both released AI models within days of each other. DeepSeek’s approach is particularly significant. It has built a high-performing AI model at a fraction of the usual costs by using reinforcement learning. This means AI development is no longer limited to organizations with billion-dollar budgets. With this shift, vertical LLMs will become more accessible, accelerating AI’s impact.
Additionally, the introduction of extremely cost-effective models is setting a new equilibrium point for the unit economics of AI. Now that the barrier is lower, a lot of the use cases that were previously cost-prohibitive are now economically viable. This shift makes AI applications more practical and affordable across industries, and will accelerate the development of specific-purpose AI agents.
This is tremendous. And while the AI race had been US-dominated we could now be witnessing a “Sputnik” moment. This AI arms race will continue and competition will be a key driver of innovation. Lower costs mean more companies can train and fine-tune models tailored to their unique needs, instead of relying on a few dominant providers. And just like the space race reshaped global power dynamics, AI is doing the same today.
Growth and the Last-Mile Problem
AI’s growth trajectory in life sciences is shifting from very early adopters to an early adoption stage, indicating that more organizations are finding effective ways to use AI. However, this expansion brings with it the last-mile problem: how do you mobilize the value to the point of value consumption or value creation?
Let’s take the example of electricity. The capabilities of AI can be likened to those of a tremendous power plant that generates a lot of brain power for different utilities. Now, the challenge becomes how to tunnel this AI power into specific business use cases that have economical value and where the parameters of these use cases align with specific industry needs.
Bridging this gap requires both a deep understanding of data science and the life sciences domain. Identifying problems with economic value and aligning AI’s capabilities to solve them are key steps. Beyond building these solutions, deploying them effectively involves change management and ensuring safeguards such as risk management frameworks.
Risk Management and the Fundamental Principle of Medicine
When deploying AI in life sciences, organizations should follow the basic principle of medicine: "first, do no harm." Risk management is essential for ensuring that AI solutions are accurate, reliable, and aligned with business needs. This involves calibrating AI outputs and tracking incidents related to AI usage. This brings with it a new set of challenges, including implementing the right cybersecurity measures, finding the right insurance, and defining the contractual terms that will allow you to do that.
In order to solve the value creation of AI in life sciences, the early adopters now need to figure out those elements. This is where AI-powered quality management and compliance systems can help connect those dots, identify solutions and provide value gained from deep domain expertise.
The Shift to Multi-Stage Processes
Recently, AI’s most common use cases involve answering questions or completing single-stage tasks. What we’re going to see in 2025 is much more agent process-oriented compared to the single conversations that we saw in 2024. The industry will see a shift to multi-stage processes managed by these intelligent agents. AI agents will take specific missions and translate them into a series of AI-driven decisions and actions. So while this evolution introduces greater complexity, it also enables broader applications.
This shift is not limited to digital workflows. AI will eventually extend from the information system layer into the physical world, particularly in areas like care delivery, pharmaceutical manufacturing, and medical device production. Robots and intelligent machines will play an increasing role, and will introduce both opportunities and new layers of complexity. Managing unstructured data and ensuring compliance with regulatory standards will become even more critical.
The Impact of a Data-Driven Culture On Decision-Making
We’ll also see the complexity of compliance with regulations and quality standards because of the amount of data that continues to grow. While many organizations are now in the process of adopting a data-driven culture, this need will be far more critical in 2025 due to the increasing speed, the volume of data and the criticality of decisions. Building this culture hinges on change management that business leaders need to consider to truly cultivate this data-driven culture.
This involves adapting business processes to high data volumes, implementing data-driven decision processes, and not just training employees but also changing their minds in a way. Everyone should truly embrace the need for a data-driven culture–because ultimately our day to day lives will be data rich, both professionally and personally.
Spreading this mindset requires leaders to rethink conventional approaches, because the conventional wisdom used in low-data environments is not necessarily the right one for high-velocity data environments. Business leaders will need to think about what this means to their organization and how they can ensure they are equipped to cope with these changes.
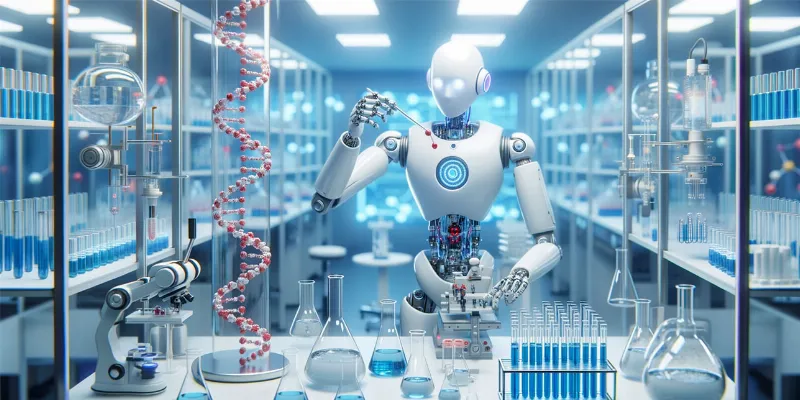
Upcoming Regulations and Addressing Bias
The regulatory landscape for AI will also evolve significantly in 2025. We’re currently in the “wild west” of AI regulation—everything goes. However, new regulatory frameworks like the EU AI Act will introduce new compliance requirements, changing how organizations deploy AI solutions. These emerging regulations will address technical aspects, such as algorithm accuracy, as well as social considerations like bias and fairness.
In the case of AI moving to the physical world, there would be new compliance issues and new challenges faced in quality. For example, if you have a robot that is taking a specific purpose or serving a specific purpose in care providing or in manufacturing, you need to analyze the way that you control or even validate those solutions. This means this new evolution of AI should also drive new methods to validate or to make sure that AI capabilities are deployed in a way that is both predictable and safe.
Bias in AI will remain a critical concern. Eliminating bias requires monitoring training data and implementing mechanisms to detect and correct biases. Organizations must also consider ethical implications, such as whether algorithms deliver consistent quality across different demographics. Proactively addressing these issues will be essential as regulators establish clearer guidelines for AI governance.
The Role of Reflection Agents
As AI becomes more sophisticated, reflection agents are gaining traction. So in the future perhaps, when we implement an AI solution, we will also implement a reflection agent that will reflect on the activities of the first agent. These multiple agents will monitor and evaluate each other, adding a layer of oversight. These reflection agents can help identify errors, meet compliance, and reduce risks, particularly in environments like life sciences.
While a human may be incapable of monitoring all of this data, AI could. This brings about an entirely new space for new AI agents that will help organizations manage risks associated with deploying AI. However, we’ll still see many in-the-loop situations where the second AI would alert a human to review the issue and adjust the decision if needed, essentially calibrating the conduct of the AI and incorporating human oversight as part of the AI-driven process.
AI and the Path Ahead
The future of AI in life sciences is unfolding fast. Companies like DeepSeek are proving that LLMs will continue to improve, becoming more accessible and powerful. This progress will bring new opportunities—but also new challenges that organizations must be ready to address.
Regulations will tighten, and expectations around AI governance will rise. Companies that stay ahead of these shifts will be in a stronger position to maintain compliance, validate AI systems, and apply the technology responsibly. The AI race isn’t just continuing—it’s accelerating. The question now is not just how AI will evolve, but how well the industry will adapt to what comes next.
Comments
No Comments Yet!