How AI Could Overcome Data Scarcity to Transform Medicine
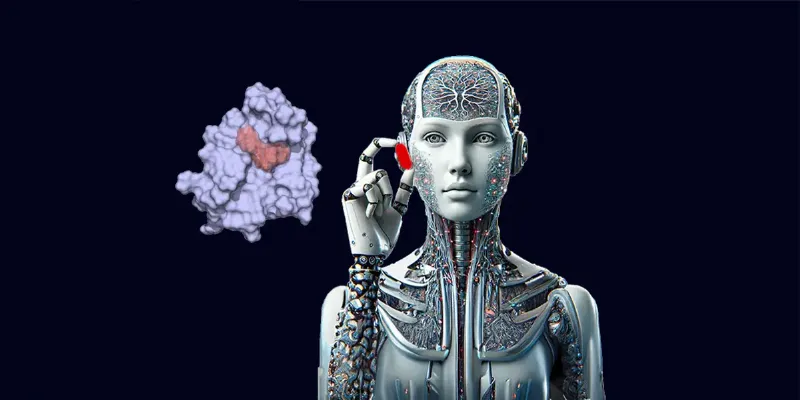
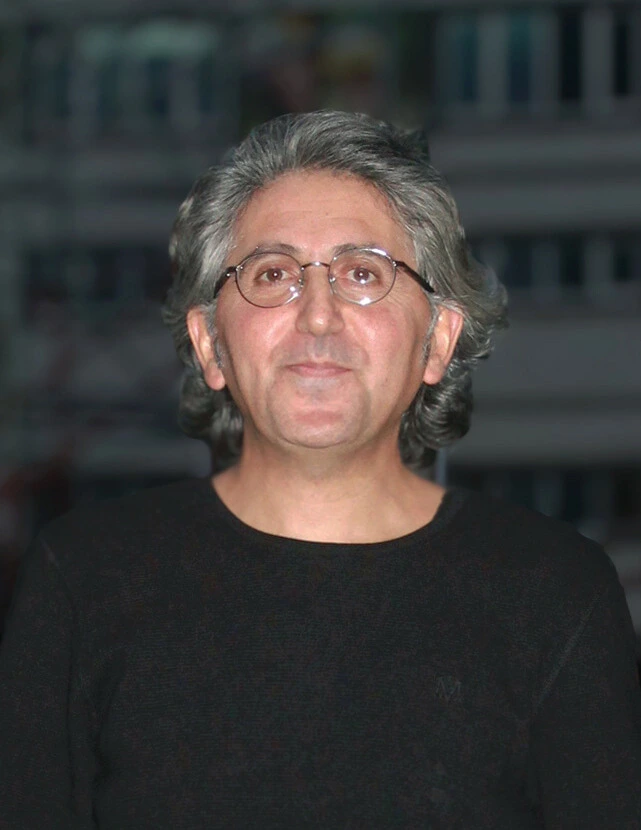
Artificial Intelligence (AI) can play a crucial role in drug discovery, but the lack of sufficient data in this area remains a significant obstacle. Verseon’s newly developed AI technology, VersAI™, elevates AI accuracy in drug discovery, even with sparse data. We recently spoke with Adityo Prakash, CEO of Verseon International, about the role of AI in the future of drug discovery and how VersAI™ is overcoming the challenges posed by sparse data.
Adityo Prakash Discuss how AI shape of drug discovery
Prakash shares insights on how Verseon’s innovative approach will redefine drug development, enhance patient outcomes, and create new opportunities for addressing rare and complex diseases.
Click the picture to view the PDF version: Pg 46-48.
VersAI™ has demonstrated significantly lower error rates compared to Google’s AutoML. Could you share more information about your new AI model? How does it work?
Prakash: While Google AutoML is the state of the art for deep learning, our proprietary AI technology uses a novel neural network architecture that’s not based on deep learning. Since we deviate from the deep learning trend, we had to create most of the underlying technology from scratch, which required significantly more effort than simply leveraging existing deep learning frameworks.
Small molecule drug discovery often deals with a vast chemical space but limited empirical data. How does your platform overcome the limitations of available empirical data?
Prakash: Over the past 150 years, humans have managed to make and test fewer than 10 million distinct chemicals for small molecule drug discovery, an insignificant fraction of the decillion (1033, or one followed by 33 zeros) compounds possible under the rules of chemistry. AI trained on such limited data can’t help us evaluate unexplored compounds.
To circumvent AI’s need for pre-existing data, we developed a series of breakthroughs in physics modeling called Deep Quantum Modeling™ (DQM™). DQM™ designs completely novel drug molecules from unexplored chemical space, predicts how they’ll bind to a protein using physics simulations, and avoids the time-consuming, costly process of making and testing every molecule in the lab.
DQM™ also generates new, synthetic data—based on completely novel molecules never before tested. Once our physics models identify promising drug structures for a disease-causing protein target, we create them in the lab and gather in vitro and in vivo data, which we then feed into VersAI™ for further training. VersAI™ is designed to learn from this sparse data with far greater accuracy than deep learning technologies like Google AutoML.
Oncology is a field where precision and accuracy in drug development are crucial. Could you discuss any ongoing or upcoming projects within Verseon’s pipeline that leverage VersAI™ to develop novel oncology therapies?
Prakash: To give one example, we’re developing small molecule drugs targeting the CD73 protein, which many tumor cells use to evade the immune system. While current efforts focus on antibody drugs, these biologics suffer from poor tumor penetration. Our first-in-class small molecule drugs will overcome this issue, offering better tumor penetration and effectiveness, making them promising immuno-oncology agents for treating a range of major cancers.
Traditional AI models require massive datasets and often struggle with predicting properties of novel drug-like structures. How does VersAI™ ensure robust predictions with much smaller datasets?
Prakash: VersAI™ was specifically developed for small, sparse datasets typical of small molecule drug discovery, and it generates better predictions than other AI methods.
It’s part of our comprehensive drug development process, which includes quantum molecular modeling and extensive lab testing. These steps provide synthetic and experimental data for VersAI™, while also verifying its predictions. The feedback from this process improves the AI models through reinforcement learning, making our drug discovery efforts more accurate and effective.
What are the future research and development plans for VersAI?
Prakash: We’re continually improving VersAI’s predictive and generative capabilities, with several advancements already in progress. Over the next few months, a series of new peer-reviewed papers will highlight recent developments in our AI technology. Our novel approach has a wi-de range of applications, and we expect to discover many new opportunities to further enhance and apply VersAI™.
AI models have been used to optimize clinical trial design and patient recruitment. How does VersAI™ contribute to this process, especially in the context of rare cancers or patient populations where data is limited?
Prakash: Rare diseases, by nature, involve small patient populations, leading to limited data. VersAI™ is better positioned than other AI technologies to analyze this limited data, helping to make predictions on trial outcomes, patient selection, and more. While each rare disease group is small, collectively, they represent a significant population.
In the future, AI will be crucial in finding solutions for these diseases. If we understand the disease mechanism, drug discovery becomes more feasible, but trials with small populations still present challenges. VersAI™ excels in making accurate predictions with limited data, adapting trials, and improving outcomes, making it invaluable in rare disease research.
As AI models like VersAI™ become more prevalent in drug discovery, what regulatory and ethical challenges do you foresee, and how are you preparing to address them?
Prakash: From an ethical perspective, how you discover drugs is irrelevant, whether you use traditional screening, conventional AI, or VersAI™ with physics modeling. There are no ethical issues with the discovery method itself. Whether the drug candidate was designed by AI or fell from the heavens, every single one must still go through the same testing process to prove its value through in vitro models, animal testing, and eventually human trials.
However, ethical concerns may arise later in the development process, particularly during clinical trials. Clinical-trials AI could introduce biases in patient selection or errors in analysis. VersAI’s real-time explainability enables researchers to rapidly evaluate and adjust the AI model as needed. It will help human researchers identify and address these issues faster, making the clinical trial process more transparent and reducing potential biases.
Looking ahead, how do you envision the role of AI in revolutionizing healthcare and drug discovery?
Prakash: It’s hard to predict the distant future, but AI has the potential to transform our understanding of human biology. When AI is combined with breakthroughs in physics and engineering, like what we’re doing at Verseon, we can generate a steady stream of new medicines to treat human diseases far more effectively than we can today.
Comments
No Comments Yet!