New AI Model VersAI™ Elevates AI Accuracy in Drug Discovery with Sparse Data
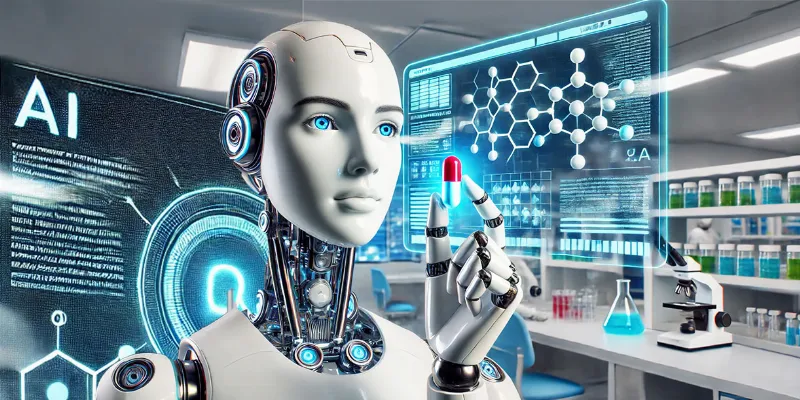
Verseon International Corporation has announced that its noval AI technology, VersAI™, demonstrates superior prediction accuracy compared to current state-of-the-art systems like Google's AutoML. Specifically designed to handle small and sparse datasets common in real-world scenarios, VersAI™ reduces AI prediction error rates by up to 35% relative to traditional deep learning frameworks.
This breakthrough could overcome the limitations of traditional AI models that require large datasets, potentially accelerating novel drug development and benefiting other fields where data scarcity hampers AI effectiveness.
The Challenge of Small Data in AI
"Most AI tools are Deep Learning frameworks, which rely on Big Data to function properly," explains Ed Ratner, Verseon's Head of Machine Learning. "This is fine when you’re training large language models on billions of webpages or when you’re training facial recognition AI on the hundreds of millions of labeled faces available on the web. But outside a handful of fields, large amounts of high-quality, dense data are often hard to come by."
This fact is especially true in many areas of the life sciences, such as small-molecule drug discovery. “The pharmaceutical industry has been hard pressed to gather enough high-quality data to create AI models that accurately predict properties of novel drugs,” said Ratner.
VersAI™: A New Approach to AI Predictions
Verseon’s effort to reduce AI prediction error rates led to the creation of VersAI™. CEO Adityo Prakash said, “Verseon has been involved in AI development long before AI was a trendy buzzword, and in recent years, we’ve pulled together a team that continues to dramatically enhance our AI capabilities.”
With typical deep learning frameworks, decreasing the error rate by just one or two percent is a difficult task that may require an order of magnitude more training data. However, on standardized benchmarking datasets with fixed sizes, VersAI™ reduces AI prediction error rates by as much as 35% compared to state-of-the-art deep learning frameworks like Google's AutoML.
“We have published peer-reviewed test results showing our lower error rates before, and we have since continued to improve on these results,” said Ratner.
Implications Beyond Drug Discovery
While VersAI™'s immediate impact is on small-molecule drug discovery, its applications extend to any field dealing with small, sparse datasets. “Beyond drug discovery, VersAI’s greater accuracy and lower error rates can dramatically improve the utility and reliability of AI in our everyday lives," Prakash adds.
How VersAI™ Changes Drug Discovery
"Deep Learning is the culmination of the 'Big Data' approach to AI," says Adityo Prakash, “It works when dense, reliable, high-quality datasets that span the entire set of possible outcomes are available to train the AI models. But such data is not available in most real-world scenarios—especially small-molecule drug discovery.”
‘’Small-molecule drug discovery is a realm of small data. The total number of possible drug-like chemical structures is a decillion (1033) or more. Yet out of that, the pharma industry has generated empirical data on fewer than 10 million chemotypes – an insignificant fraction of the total space – over the past 150 years. Even within that tiny pool, the data is not that dense or reliable, nor is it consistently high quality.
In this context, Deep Learning often struggles to predict the properties of drug-like structures at all. And the results only get worse farther outside the boundaries of the available training dataset. Consequently, current AI has not lived up to its promise and has mostly produced small tweaks on known molecules, with most failing in trials or being shelved midway through development. Deep Learning and other approaches that require large volumes of training data have a “data problem.”
Verseon sidesteps this data problem with its fundamental breakthroughs in physics modeling that do not require preexisting training data. The predictions from Verseon’s model are sufficiently accurate that its results can be used as what data scientists call “synthetic data.” Furthermore, Verseon makes the modeled compounds in the lab and develops additional in vitro and in vivo empirical data.
However, this new empirical data is still sparse. So, Verseon’s team developed VersAI™ to handle small, sparse data. Its unique, stochastic non-iterative algorithm requires far less data than Deep Learning to produce actionable results. And as Verseon’s published peer-reviewed results demonstrate, VersAI™ does so with much greater accuracy. Once Verseon’s physics modeling platform designs a set of promising chemotypes for a drug program, VersAI™ optimizes the initial set of drug candidates using the sparse data available.
For every one of its drug programs, Verseon’s physics-modeling and VersAI™ technologies working together systematically design multiple novel drug candidates that cannot be found by any other method, including other AI-based approaches. Each of Verseon’s drug candidates possesses uniquely desirable therapeutic profiles and promises to change the standard of care for the disease it addresses. Using the right combination of physics, AI, and other tools to bring better therapeutic options to market will fundamentally change what patients can expect from medicine.’’
Comments
No Comments Yet!