New AI Model Detects Antibiotic Resistance with Over 80% Accuracy
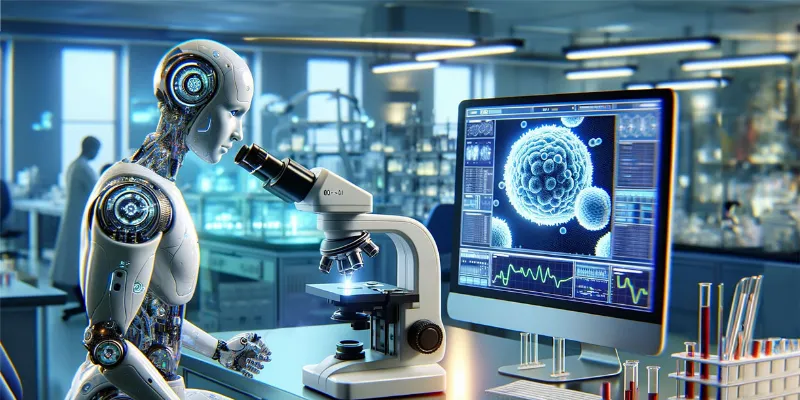
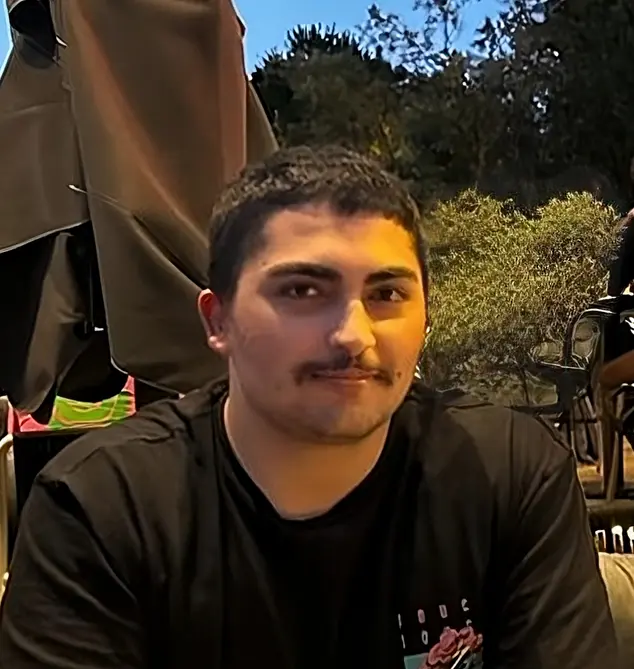
Digital Health |
24 November 2023
In a groundbreaking study by Oxford University, a new AI model was used to detect antibiotic resistance in bacteria within 30 minutes, achieving over 80% accuracy. This represents a significant improvement over current methods, which require several days. The rapid approach could revolutionize treatment, reducing side effects and combating the rise of antimicrobial resistance.
The study, published in Communications Biology, introduces a unique AI method that combines fluorescence microscopy and AI to analyze bacterial cells. It offers swift detection of antimicrobial resistance (AMR), a top global health threat that caused about 1.3 million deaths in 2019. This innovation promises more accurate and prompt treatments for infectious diseases, potentially saving lives.
Currently, the 'gold-standard' testing for AMR involves growing bacterial colonies in antibiotics, a slow process often unsuitable for urgent medical situations. The new method from the Oxford Martin Programme (OMP) offers a promising solution to this issue.
''In an era where AMR poses a critical public health threat, our team has made a ground-breaking advancement toward rapid detection of AMR. This innovation may hold the potential to revolutionise the way we respond to infectious diseases, allowing for more precise and timely treatment decisions, ultimately saving lives,'' said Co-author Dr Piers Turner, OMP on AMR Testing at the University of Oxford.
The AI-based technique focuses on detecting structural changes in bacterial cells when treated with antibiotics, specifically in Escherichia coli (E. coli). It employs deep-learning models to analyze these changes with impressive efficiency, achieving at least 80% accuracy on a per-cell basis within just 30 minutes. This approach was effective across multiple antibiotics, including ciprofloxacin, gentamicin, rifampicin, and co-amoxiclav.
Dr. Piers Turner and Professor Achillefs Kapanidis, leading the research team, emphasize the groundbreaking nature of this advancement. Their method can differentiate between resistant and non-resistant bacterial cells, providing a basis for detecting AMR. This rapid detection is not only significantly faster than established methods but also holds promise for a range of antibiotics and bacteria types.
The team's goal is to further develop this method for broader clinical application, making it faster and more scalable. This could lead to more targeted antibiotic treatments, reducing treatment times, minimizing side effects, and, crucially, slowing down the rise of AMR.
This breakthrough AI model represents a hopeful stride forward in the global fight against AMR, offering a glimpse into a future where rapid, accurate detection can transform the way we tackle one of the greatest public health challenges of our time.
Abstract of the research
Deep learning and single-cell phenotyping for rapid antimicrobial susceptibility detection in Escherichia coli
Abstract: The rise of antimicrobial resistance (AMR) is one of the greatest public health challenges, already causing up to 1.2 million deaths annually and rising. Current culture-based turnaround times for bacterial identification in clinical samples and antimicrobial susceptibility testing (AST) are typically 18–24 h. We present a novel proof-of-concept methodological advance in susceptibility testing based on the deep-learning of single-cell specific morphological phenotypes directly associated with antimicrobial susceptibility in Escherichia coli. Our models can reliably (80% single-cell accuracy) classify untreated and treated susceptible cells for a lab-reference fully susceptible E. coli strain, across four antibiotics (ciprofloxacin, gentamicin, rifampicin and co-amoxiclav). For ciprofloxacin, we demonstrate our models reveal significant (p < 0.001) differences between bacterial cell populations affected and unaffected by antibiotic treatment, and show that given treatment with a fixed concentration of 10 mg/L over 30 min these phenotypic effects correlate with clinical susceptibility defined by established clinical breakpoints. Deploying our approach on cell populations from six E. coli strains obtained from human bloodstream infections with varying degrees of ciprofloxacin resistance and treated with a range of ciprofloxacin concentrations, we show single-cell phenotyping has the potential to provide equivalent information to growth-based AST assays, but in as little as 30 min.
Comments
No Comments Yet!