AI-Supported Screening Tool Revolutionizing Early Autism Detection
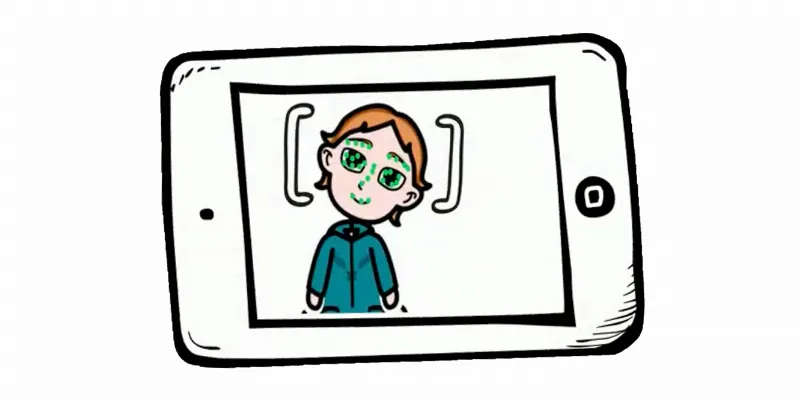
10 October 2023
A new digital screening tool, developed with the support of the National Institutes of Health, could revolutionize early detection of autism spectrum disorder, particularly among underdiagnosed groups such as girls and children of color. This AI-supported app correctly identified most cases of autism with 87.8% sensitivity, according to a new study published in Nature Medicine.
The SenseToKnow app, available for phones and tablets, displays short videos to children and captures their reactions using the device's camera. The app uses computer vision analysis to automatically detect early signs of autism from these reactions, such as gaze patterns, facial expressions, and head movements. The data is then processed using machine learning, resulting in an artificial intelligence-based algorithm capable of screening for detection of autism spectrum disorder (ASD).
“We need screening tools based on direct observation of the child's behavior that are objective and scalable. Early detection is important because providing early intervention has a significant impact on children's outcomes,” said Professor Geraldine Dawson.
Additionally, a bubble-popping game within the app evaluates motor skills, another indicator of autism, as highlighted by Professor Dawson.
During the study, healthcare providers utilized the SenseToKnow app to screen 475 toddlers aged 17 months to 3 years during regular child wellness visits. The results were promising: the app demonstrated an 87.8% sensitivity in detecting ASD, meaning it correctly identified a significant majority of children with the condition. Its specificity, or the percentage of children without ASD who were correctly identified as not having the disorder, stood at 80.8%.
Compared to the standard parent questionnaire method, which has a detection rate of about 15%, toddlers screened with the SenseToKnow app, had a 40.6% likelihood of being diagnosed with ASD at a later stage. Interestingly, combining both the app and the traditional questionnaire method improved diagnostic outcomes further, with a 63.4% probability of accurate detection.
The team is now working on validating the app's ability to monitor a child's behavior over time, which could be pivotal in assessing the efficacy of certain therapies. Professor Dawson expressed hope that, in the future, primary healthcare providers will use both parent reports and objective tools like SenseToKnow to make more informed decisions regarding referrals for further diagnostic evaluations.
This groundbreaking research, published in Nature Medicine, underlines the potential of technology in addressing disparities in early autism detection and intervention. With the backing of institutions like NIH’s Eunice Kennedy Shriver National Institute of Child Health and Human Development and the National Institute of Mental Health, digital tools like the SenseToKnow app may soon become standard in pediatric care.
Abstract of the research
Early detection of autism using digital behavioral phenotyping
Abstract: Early detection of autism, a neurodevelopmental condition associated with challenges in social communication, ensures timely access to intervention. Autism screening questionnaires have been shown to have lower accuracy when used in real-world settings, such as primary care, as compared to research studies, particularly for children of color and girls. Here we report findings from a multiclinic, prospective study assessing the accuracy of an autism screening digital application (app) administered during a pediatric well-child visit to 475 (17–36 months old) children (269 boys and 206 girls), of which 49 were diagnosed with autism and 98 were diagnosed with developmental delay without autism. The app displayed stimuli that elicited behavioral signs of autism, quantified using computer vision and machine learning. An algorithm combining multiple digital phenotypes showed high diagnostic accuracy with the area under the receiver operating characteristic curve = 0.90, sensitivity = 87.8%, specificity = 80.8%, negative predictive value = 97.8% and positive predictive value = 40.6%. The algorithm had similar sensitivity performance across subgroups as defined by sex, race and ethnicity. These results demonstrate the potential for digital phenotyping to provide an objective, scalable approach to autism screening in real-world settings. Moreover, combining results from digital phenotyping and caregiver questionnaires may increase autism screening accuracy and help reduce disparities in access to diagnosis and intervention.
Comments
No Comments Yet!