AI Shows Equal Accuracy as Radiologists in Breast Cancer Screening
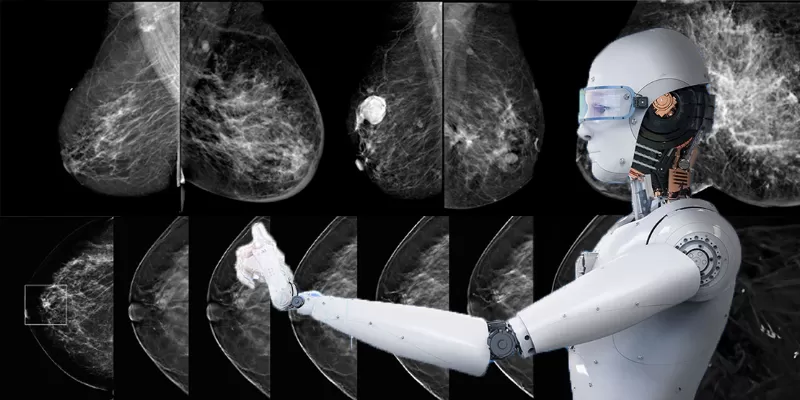
5 September 2023
A groundbreaking study led by the University of Nottingham has shown that artificial intelligence (AI) can interpret challenging mammogram images as accurately as expert human readers. Currently, many medical institutions use a "double read" approach, where two experts review each mammogram to enhance accuracy. This study, published in Radiology, evaluated whether AI could replace the second reader.
The results revealed that the AI matched human performance, indicating its potential in streamlining breast cancer screening. The results, recently published in the medical journal Radiology, could have significant implications for the future of breast cancer screening. Breast cancer screening through mammograms is an essential tool for detecting early signs of the disease.
However, its effectiveness has limitations. Sometimes, the screenings can miss cancer cases or yield false positives, causing unnecessary biopsies. To mitigate these inaccuracies, many medical institutions adopt a "double read" approach: two experts examine each mammogram. While this method increases cancer detection rates and decreases false positives, it requires substantial time and resources.
The researchers, under the leadership of Dr. Yan Chen, professor of digital screening at the University of Nottingham, aimed to determine if artificial intelligence could potentially replace the second human reader, thus streamlining the process.
Research team used test sets from the Personal Performance in Mammographic Screening scheme, quality assurance assessment utilized by the UK's National Health Service Breast Screening Program, to compare the performance of human readers with AI. The sample comprised 60 images deemed "challenging" that included 161 normal results, 70 indicating signs of cancer, and nine showing benign growths.
"It's really important that human readers working in breast cancer screening demonstrate satisfactory performance. The same will be true for AI once it enters clinical practice. The 552 readers in our study represent 68% of readers in NHSBSP, so this provides a robust performance comparison between human and AI. The results of this study provide strong supporting evidence that AI for breast cancer screening can perform as well as human readers," Prof. Chen said.
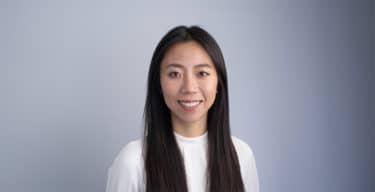
When benchmarked against the average human performance metrics of 90% sensitivity (the ability to correctly identify positive cases) and 76% specificity (the ability to correctly identify negative cases), the AI algorithm demonstrated a sensitivity of 91% and a specificity of 77%. Essentially, the AI system was able to match the performance of human readers.
More research is needed before AI can be used as a second reader
Prof. Chen also pointed out the necessity of continuous AI performance monitoring, noting that algorithms can degrade over time or be influenced by changes in operational environments. The study, which evaluated AI against the performance of 552 human readers, represents a milestone as no other research has made such extensive comparative assessments.
This achievement sets a potential standard for gauging AI performance in real-world settings. In summary, while AI presents a promising tool in enhancing breast cancer detection, a measured approach is crucial. With extensive research and the right monitoring mechanisms, AI could play an essential role in the evolution of mammographic screening.
Abstract of the research
Performance of a Breast Cancer Detection AI Algorithm Using the Personal Performance in Mammographic Screening Scheme
Key Results: No difference in performance was observed between artificial intelligence (AI) and 552 human readers in the detection of breast cancer in 120 examinations from two Personal Performance in Mammographic Screening test sets (area under the receiver operating characteristic curve, 0.93 and 0.88, respectively; P = .15). When using AI score recall thresholds that matched mean human reader performance (90% sensitivity, 76% specificity), AI showed no difference in sensitivity (91%, P = .73) or specificity (77%, P = .85) compared with human readers. Background: The Personal Performance in Mammographic Screening (PERFORMS) scheme is used to assess reader performance. Whether this scheme can assess the performance of artificial intelligence (AI) algorithms is unknown. Purpose: To compare the performance of human readers and a commercially available AI algorithm interpreting PERFORMS test sets. Materials and Methods: In this retrospective study, two PERFORMS test sets, each consisting of 60 challenging cases, were evaluated by human readers between May 2018 and March 2021 and were evaluated by an AI algorithm in 2022. AI considered each breast separately, assigning a suspicion of malignancy score to features detected. Performance was assessed using the highest score per breast. Performance metrics, including sensitivity, specificity, and area under the receiver operating characteristic curve (AUC), were calculated for AI and humans. The study was powered to detect a medium-sized effect (odds ratio, 3.5 or 0.29) for sensitivity. Results: A total of 552 human readers interpreted both PERFORMS test sets, consisting of 161 normal breasts, 70 malignant breasts, and nine benign breasts. No difference was observed at the breast level between the AUC for AI and the AUC for human readers (0.93% and 0.88%, respectively; P = .15). When using the developer's suggested recall score threshold, no difference was observed for AI versus human reader sensitivity (84% and 90%, respectively; P = .34), but the specificity of AI was higher (89%) than that of the human readers (76%, P = .003). However, it was not possible to demonstrate equivalence due to the size of the test sets. When using recall thresholds to match mean human reader performance (90% sensitivity, 76% specificity), AI showed no differences inperformance, with a sensitivity of 91% (P =. 73) and a specificity of 77% (P = .85). Conclusion: Diagnostic performance of AI was comparable with that of the average human reader when evaluating cases from two enriched test sets from the PERFORMS scheme.
Comments
No Comments Yet!